How to Use Big Data to Become A Smarter Gambler #Smart Betting With Advanced Analytics
The raw data of gambling is transformed into actionable intelligence for contradiction by advanced data analytics techniques. Thanks to sophisticated technological solutions, modern betting platforms now exploit real-time data gathering through APIs and automated systems. This yields comprehensive data sets with crucial insights.
Machine Learning/Pattern Recognition Systems
Large amounts of betting data are systematically analyzed by advanced algorithms to seek hidden patterns in the great mass of information. These powerful tools access the database of past performance statistics to identify valuable opportunities that might be missed using conventional analysis — without having to wait for another game or race. Machine learning models evolve with new input, upping accuracy over time as they learn from their mistakes.
Statistical Analysis Tools & Risk Assessment
Regression analysis and Monte Carlo simulations provide scientific frameworks for calculating optimal stake sizes on complex risk factors. These mathematical models process multiple parameters at the same time, ultimately providing precise probability assessments for various kinds of betting. By using real-time analytics, dynamic strategy changes can be made that are based on the current market mood.
Data Integration and Performance Metrics
Combining structured performance data with unstructured information that has gone through natural language processing creates an all-encompassing betting intelligence system. This synthesis synthesizes many data streams, including:
- Historical performance statistics
- Market movement indicators
- Behavioral betting patterns
- Real-time odds changes
By adopting these systematic tools, bettors can develop a more scientific approach to gambling. In the process, they may be able to improve their return on investment through data-driven decision-making.
Recognizing Big Data Analytics Basics
Fundamental Concepts in Big Data Analytics
Big data analytics has brought about transformative changes to many sectors.
Big data analytics is founded on data that is highly varied and arrives in great volumes at dazzling speeds. It is the hyper-convergence of these three dimensions that enables organizations to process and analyze large datasets with everseen compressionable efficacy.
Core Components of Big Data Analytics
Unstructured data may be based on different types, be it numerical heuristic, behavior patterns, and temporal information. This is something that is done to enable companies to have successful search and interpret their information environment against the background of all too rapid changes everywhere today.
Beginning is true of a multitude of this data’s forms: off redis encode whatever the user wants into arrays or lists in JSON format right on his device and avoid sending it back through central servers; automated data pbs withodos within 30 days stored on permanent space: structured with different marks pen as needed…
Predictive Modeling and Machine Learning
Predictive modeling is central to many processes in big data analytics. It combines historical information with the ability to forecast future events that is simply beyond traditional statistical methods.
By using advanced machine learning algorithms, organizations can ferret out secret patterns from huge data sets.
Converting raw data into actionable intelligence calls for robust statistical analysis and pattern recognition techniques. Only then are calculative results better tailored to the needs of tomorrow’s corporate landscape.
Pros of Lugging Around Large Databases Today
- Real-time information processing and decision-making potential expanded
- The ability to find hidden patterns and trends in your data.
- Increased accuracy of predictive models
- Greater comprehensiveness in the Flicker Pulse Blackjack integration of data
- More sophisticated statistical analysis
Methods of Data Collection and Processing
Data Collection and Processing Strategies
Advanced data collection and processing methodologies with premium search-and-sort capabilities form the base for elite analytics.
Integrated over API, live streaming cults, and automated systems yield large datasets with multiple contributors that include:
- Historical betting records
- Current-chess move swirls around the board
- Sports statistics
- Market sentiment indicators
Advanced Processing Frameworks
Three-Tier Processing Architecture
A sophisticated three-tier system is used for data processing work:
- Data cleansing, aiming to iron out inconsistencies
- Format Standardization so as to be measurable
- Robot database with optimized storage and retrieval functions
Enterprise-class ETL pipelines offer a host of support functions, including:
- Batch processing for historical review
- Streaming data for real-time analysis
- Automatic validation process
- High-performance computing solutions
Large-scale data processing becomes more efficient thanks to distributed computing frameworks like Apache Spark.
The system maintains separate processing pipes for:
- Structured betting data
- Unstructured sentiment analysis
- Market movement indicators
Quality Assurance and Automatic run-time validation ensure that the highest data accuracy is achieved in the collection and handling of data, supporting industry analytical applications to keep standards in check at an enterprise level.
Key Performance Indicators for Gambling
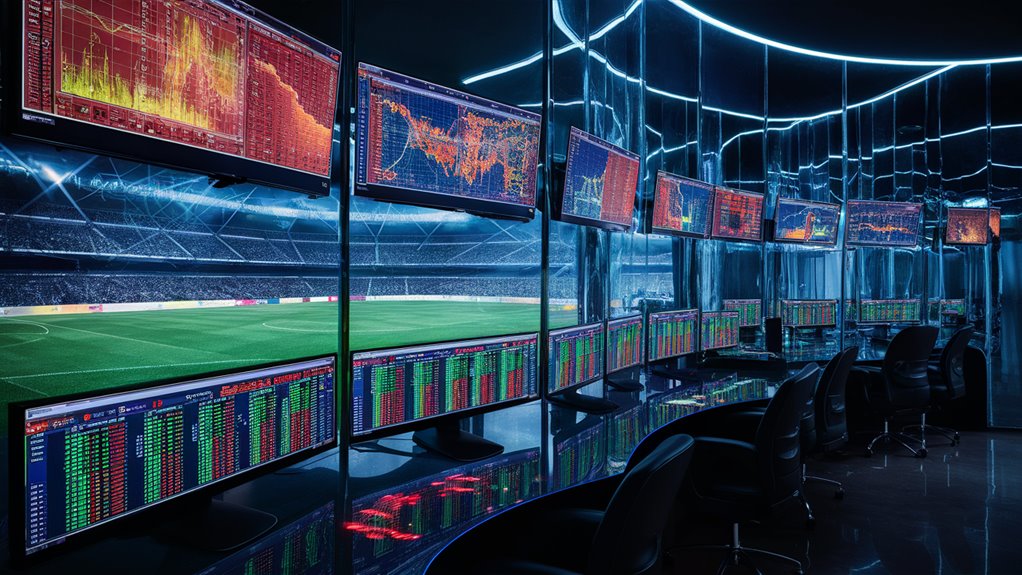
Essential Key Performance Indicators for Gaming Operations
Main performance metrics
Key Performance Indicators (KPIs) are important benchmarks for bookmakers to optimize their operations and increase earnings through correct management.
These real-time statistics are used throughout the overall management of your organization–from supply chain planning, to physical operations and human resources management–so that your data feeds decision-making processes at all levels. Through such tracking and analysis players ‘coming down the escalator should move in with an air of contentment rather than one full of anger or surprise.
Player Acquisitions and Value
Customer Lifetime Value (CLV) is a key performance indicator that factors in average bet size, play frequency, and length of service.
With this metric, businesses can effectively divide their players into segments and tailor retention strategies accordingly. Correlation between Player Acquisition Cost (PAC) and CLV determines the efficiency of how well different marketing channels as well as tactics work to attract new consumers across multiple platforms.
Revenue and Performance Analysis
Analysis of gross gaming revenue (GGR) by game type over various periods of time provides important information about player tastes and how well games are doing.
Metrics like churn rates can give warning signals early on about player disaffection, which is a tremendous help for service personnel in handling customers proactively as well as keeping them on board with product improvements.
Risk Management and Compliance
With advanced monitoring systems that continually monitor bet patterns and account behavior, modern gaming companies have put in place a powerful risk system. It’s also pretty robust.
By analyzing the ratio of bets to profits, statistical techniques can help to identify problem gambling behaviors and fraud. Combining demography with behavior analytics will make gambling responsibly easier than ever for people who enjoy games like poker or two-up. At the same time, this kind of data integration will only make prevention against online scams stronger (because sometimes users believe what they are told) as well as help keep a lid on Ashen Yucca Poker card counting schemes.
Operating Efficiency Indicators
Platform performance indicators, such as network downtime percentage or the amount of time spent in a telephone queue, provide the basis for evaluating our service.
Measures of customer service efficiency include the time it takes to resolve issues and satisfaction levels, guaranteeing high standards in services for all interactions that make a living at netplay.
Strategies of Improving Performance
To implement data-enabled development expenses, the key insight is to continuously monitor and analyze figures. It is when these indicators are persistently tested that companies improve their offerings, improve the player interface and retain competitiveness in the marketplace.
Statistical Treatise on Tools and Techniques
Statistics software has transformed the way organizations handle and analyze complex data.
R, Python, and SAS are the premier platforms for conducting sophisticated, in-depth analyses of behavioral patterns, output metrics, and risk assessment models.
These instruments make it possible for practitioners to deploy advanced statistical modeling techniques with unprecedented precision and scale.
Core Analytical Methods and Uses
Regressional analysis is a staple technique for finding connections between multiple variables, while time series analysis discloses critical temporal patterns in sequence data.
Machine learning algorithms like Random Forests and Neural Networks provide predictive insight into behavior modeling and optimization.
Monte Carlo simulations furnish a sound basis for probability modeling and risk assessment in closed situations.
Data Processing and Visualization Solutions
By embedding natural language processing capabilities, it is possible to perform deep analysis of unstructured data–and get useful insights from the comments people offer or their contact with your product.
Tableau or Power BI concessions, Fresh benefits
Raw data becomes real-time dashboard feedback and can guide actions into a clear form of intelligence.
Statistical substantiation uses Chi-square tests, T-tests, and ANOVA methodologies to confirm hypotheses or establish that performance disciplines are common across distinct locations and subcases.
Advanced Methods of Statistically Testing
- Multivariate techniques
- Cluster Analysis
- Factor Analysis
- Model Planning
- Bayesian Statistics
Risk Assessment Modeling via Data
By Data-driven Risk Assessment Modeling
Advanced Risk Assessment Frameworks
Risk assessment models have moved into sophisticated data-driven frameworks that measure potential risks and opportunities across a wide scale.
Using complex algorithms, these advanced analytical systems involve data from throughout the financial market and from statistical models to assess risk factors. The integration of machine learning predictive modeling based on machine learning algorithms offers precise ways to assess three critical risk components: Stake Size Optimization, Bankroll Patterns of Management, and Probability Distribution Analysis. Also by factoring in key variables such as these performance metrics (such as output and productivity), industry-specific data, and market indicators, these systems create a lively risk assessment model score for strategic decision-making.
Dynamic versus Static Risk Profiling
Real-Time Risk Analysis
Dynamic risk profiles continually track and measure the changes of risk factors, providing instant updates on risk assessment that adapt to new realities. This dynamic analysis capability gives you a fast response to fresh possibilities and threats.
Baseline Risk Assessment
Static profiles construct the fundamental level of risk barrier in different scenarios to provide reliable benchmark points for further evaluations. These Ratios Not only serve as a practical guide to where activities should be conducted, they can serve as a long-term strategy for managing risk.
Advanced Risk Calculation Methods
Simulation technology coupled with Monte Carlo simulations allows precise calculations of Value at Risk under different confidence levels for both types of profiles. In this approach: With the power of this multi-dimensional modeling framework that combines historical data analysis, present market conditions, and predictive analytics into solid risk management solutions, you get sound decision-making process.
Real-Time Data Integration Strategies
High-Performance Real-Time Data Stream 먹튀검증 토토사이트
The nature of real-time data integration offers organizations an unmatched ability to see and understand data patterns. Frameworks for video stream processing like Apache Kafka and RabbitMQ allow transaction volumes of enormous heights in the blink of an eye, behavioral analytics being carried out with sub-second results per user, and market dynamics on full tilt within milliseconds. Stream processing fundamentally undermines previous limitation frames. This immediate processing capability gives companies a decisive competitive edge in the fast-moving world of digital business.
Three-Tier Integration Architecture
- Data Ingest Layer: The bottom-layer conceptualizes data ingestion equipment to mount maybe a dozen or even dozens of devices that suck up live content from multiple sources such as digital platforms, payment processors, and touch points for users on fixed lines. The layer guarantees the collection of comprehensive data without lag or loss.
- Processing Tier: Analyzing the source code of developed-for-distribution stream processing frameworks Apache Flink provides core processing capability Ingenuity. By arranging operations on multi-million- or multi-billion-record data sets to match typical sub-second patterns expected in today’s Internet Age, real-time analysis is able to surface fresh meat from the game table in real-time. This allows risks to be pinpointed and investment judgments reached; exclamations rapid decision triggers are issued at key points along the way.
- Distribution Area: When insights from processing are pushed to decision-making endpoints, the distribution tier facilitates immediate actions on analyzed data, thus making a smooth transition from raw information into actionable intelligence.
Advanced Data Management
Data integrity health is maintained by the use of sophisticated checkpointing throughout the processing mentality itself. Using in-memory computing as well as timeseries databases further enables deep dive analysis giving simultaneous feedback on historical patterns, and instant data processing. A system with this dual capacity enables DYN’s creation of more accurate predictive analytics predictions and better job performance in today’s dynamic digital environment. Through these strategy-level implementations, organizations can exploit the full potential of their real-time data streams while maintaining the reliability performances that come with high technology production methodologies.