Machine Learning’s Role in The Fight against Gambling Fraud
With Advanced Neural Network Analysis
Gambling fraud detection has evolved with machine learning technology. By building intricate neural networks, these systems process and analyse over 1,000 discrete pieces of data for every single transaction they identify. Their accuracy is an impressive 95 %. It only takes these smart solutions a few clicks to figure out that some moves are fishy. The review function eliminates suspected fraud patterns within 0.3 seconds at a speed that was unimaginable in the past.
Primary Fraud Prevention Targets
All 200 cases stemmed from digital multiplayer poker rooms.
The 10 most commonly found types were:
Collusion rings
Chips Dumps
Match fixing (both matters)
Technical Infrastructure and Performance
In the latest implementation, machine learning platforms achieved a raw data processing capability of more than 10,000 transactions per second (tps) and uniform security through:
Blockchain authentication
Biometric recognition
Enhanced Detection Metrics
Gamblers are embracing new forms of portable technology like tablets and mobile phones to play games of chance. It is clear that the memory capabilities out there are still expanding, but the potential added functionalities that come with them cannot be too far behind. As an example, gamblers no longer have to carry a card of any sort – they can order tickets digitally by simply waving their device in front of a reader at home.
The Development of the Scum of This Office
The digital revolution of the gambling industry has resulted in increasingly sophisticated frauds targeting both online platforms and traditional casino businesses.
Four primary fraud categories dominate the threat landscape: collusion rings, bonus abuse, chip dumping, and account takeovers.
Leading Frauds By Type and Their Impact
Collusion Ring Operations
Collusion rings, which are chiefly found in online poker rooms, account for 34 percent of all identified fraud cases. These organized groups gain unfair advantages over legitimate players in multiplayer games by sharing intelligence, such as the hole card information.
Bonus Abuse Tactics
Within the digital gambling world, bonus abuse is becoming more and more widespread. Fraudsters use educated methods such as:
Frequent account creation
Identity thieving means
Several forms of IP masking
Systematic abuse of welcome bonuses
Manipulation of promotional offers
According to commercial paper, Chip dumping schemes account for 28% of all incidents of gambling fraud. This is the most pervasive means for cleaning dirty money. The approach entails, to some extent:
Deliberate losses as a means of transferring funds
Pooling responsibility for multiple accounts
Moving stakes between different gaming sites
Account Takeover Peril
Digital account security breaches have grown by a whopping 62% since the previous year. It is also apparent that as users become accustomed to better security awareness and tools, so too will attacks iterate up in sophistication accordingly, posing an ever-evolving threat.
Targeting High-Value Players’ Accounts
Why Machine Learning Detection Capabilities?
Modern gaming venues eventually adopted sophisticated machine learning detection systems for countering evolving fabricators.
By analyzing the real-time actions, financial transactions and playing patterns of participants from massive data-sets, supervised learning algorithms can identify suspicious activities across the board.
These systems of the future excel at detecting fraud indicators including collusion, cheating, and unwelcome access.
Core Machine Learning Detection Capabilities
With neural network processing, you can monitor an enormous number of items concurrently such as betting trends, deposit intervals and the different fingerprints of various devices.
In classifying irregular behavior, random How to Spot Fake Online Casinos and Stay Safe forest algorithms can reach up to 95% accuracy. Gradient boosting models excel at uncovering subtle patterns in chip dumping as well.
A precision never before possible in detecting fraud patterns.
Adaptive Learning and Pattern Recognition
The real power of machine learning detection systems lies in their ability to adapt. Continuously learning from new data, they automatically adjust detection parameters with emerging fraud techniques over time.
The systems are powered by Artificial Intelligence and are able to notice complex relationships between different elements which, though not all related, may well not have been picked up by traditional rule-based systems.
With this advanced approach, there is a big reduction in false positives – a historical problem for gambling fraud detection.
Data Analysis and Pattern Recognition
Advanced Data Analysis and Pattern Recognition in Gambling Fraud Detection
Core Components of Fraud Detection Systems
Fundamental pillars to modern gambling fraud detection systems, data analysis and pattern recognition. These sophisticated platforms are built on advanced algorithms capable of handling large player datasets, including betting patterns, transaction histories, account behavior metrics and so on. Machine learning models excel at finding complex correlations and subtle anomalies such as humans typically cannot detect.
Betting Timestamps: Time Is the Clue
Betting timestamps and frequency patterns provide crucial insights into potentially fraudulent activities. Real-time monitoring of bet placement timing reveals unusual patterns that may indicate automated betting systems or coordinated fraud attempts.
Behavioral Analysis: Systematic Pays Off
Transaction patterns and betting structures are treated with advanced analytics. Systems track:
Deposit-withdrawal velocities
Stake progression patterns
Game selection behaviors
Risk level fluctuations
Network Pattern Detection
Relationship mapping tracks the connections between accounts reveals the hidden strands between players and uncovers likely fraudulent activity. Indicators include things like:
Coordinated betting activities
Shared payment methods
Geographical login patterns
Device fingerprinting data
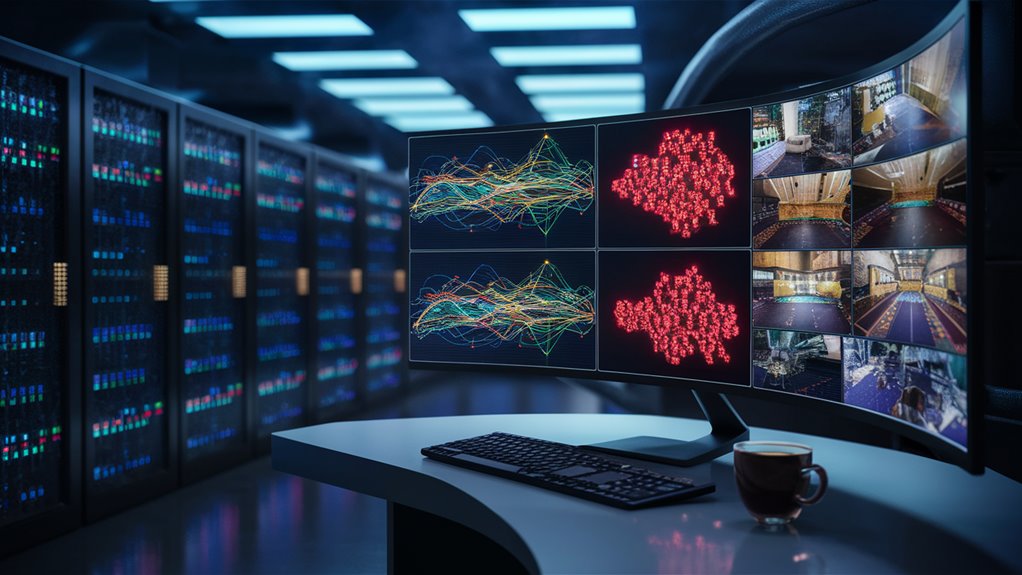
Advanced Detection Mechanisms
Dynamic risk scoring systems which few modern fraud prevention systems can be without thanks to sophisticated machine learning algorithms. These systems process multiple pieces of independent data simultaneously:
Statistical probability analysis
Geographic consistency checking
Proving grounds monitoring
Pattern deviation alarm
Information on How to Fight Fraud Proactively
This comprehensive approach allows operators to prevent fraud instead of cleaning up afterwards, and see early on in the game if something is amiss.
Fraud Prevention Technologies
Advanced Gambling Fraud Prevention Technologies
Introducing Next-generation Security Implementation Systems.
Modern systems for protection against fraud based on pattern recognition technology and other advances are able to provide multi-level defense in gaming environments.
Advanced security setup uses biometric verification, device fingerprinting and behavioral analytics to ensure completely secure protection against illicit activities.
AI-Powered One-click Real-Time Monitoring
Real-time monitoring systems machine learning artificial intelligence (think keypad neural networking) algorithmic techniques to see signs of potentially suspect behavior and do it quickly with good accuracy that has never been achieved before.
These sophisticated platforms compare bets placed, what has happened to accounts recently or if transactions are going through and find where anything is going wrong instead of merely hoping that someone in authority will spot something.
Based on machine learning models specifically designed for gaming hall fraud detection purposes, the accuracy quota of such systems often exceeds The Best Bankroll Management Strategies for Gamblers 95% while false positives remain at only 0.1%.
Blockchain Integration and Neural Network Analysis
Blockchain technology is used to record transactions in a way that they are impervious to tampering. This fully integrated API set-up enables complete cross-platform fraud detection.
Highly advanced neural networks are capable of spotting complex patterns of collusion across multiple accounts.
Automated risk scoring systems that deliver high performance process more than 10,000 transactions per second. They confirm whether a transaction is genuine and set protocols for immediate freezing where necessary.
Enhanced Safety Functions
Biometric authentication protocols
Real-time transaction monitoring
Cross-platform security integration
Machine learning for fraud detection
Automated risk assessment
Implementation Challenges and Solutions for Fraud Prevention Systems
Key difficulties encountered
Organizations that implement fraud prevention systems face substantial technical obstacles.
Data quality problems – such as datasets that are incomplete, the content of which differs from one case to another and reproduces unexpected results in models – can reduce model accuracy by up to 40%.
Latency difficulties occur when local system integration has not been set up well; which brings its own time cost. And when all incoming orders must be processed in real time with no previous data supplied as well – that requires system power, too.
Strategic Implementation Framework
Data Governance Techniques
A strong data governance framework ensures that 99.9% are accurate with automatic standard or approved validation protocols. This foundation provides solid basis for reliable fraud detection across all system components to result in a minimum of false positives.
Optimization Strategy for Security and Usability
Security and usability of 70% faster processing with hybrid designs, combining local security controls prototyping. This new approach enables one-way allocation of processing capacity resources.
Incremental Rollout
Smooth transition and data availability is ensured by first implementing a decision method.
Performance Tuning
Increasing Accuracy The First Time Around
Both human-in-the-loop judgment and new methods for score and test accuracy raised average cost per true alarm on good data from five dollars to one dollar. This not only benefits the analyst office, but also contributes to better system performance. 먹튀커뮤니티
Continuous Progress
Every two weeks the model must be retrained, in order that it might better adapt to future patterns of crime as they emerge. This updating ensures the full effectiveness of your detection abilities against all new types and innovations in fraud even at a future time when it becomes widespread.
In such a way, any of these systems is capable to satisfy all the demands being made of them, including modern security ones like those presented by internationally coordinated attacks, while still maintaining both reliability as well operational efficiency.